Unifying Structure and Activation: A Comprehensive Approach of Parameter and Memory Efficient Transfer Learning
Mar 3, 2025ยท
,,,ยท
1 min read
Jin Tian

Enjun Du
Changwei Wang
Wenhao Xu
Luo Ding
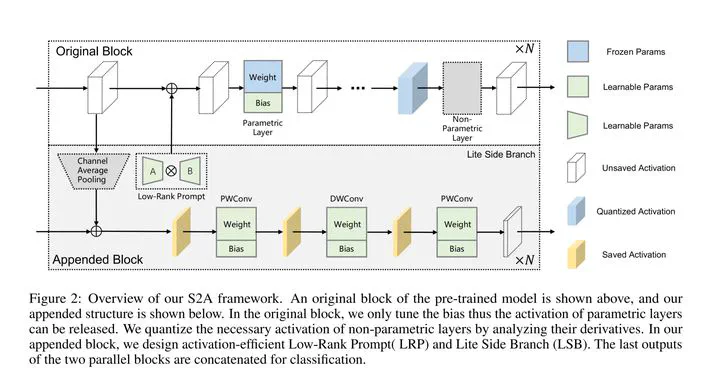
Abstract
Parameter-efficient transfer learning (PETL) aims to reduce the scales of pre-trained models for multiple downstream tasks. However, the memory footprint of existing PETL methods is not significantly reduced compared to the reduction of learnable parameters. This limitation hinders the practical deployment of PETL methods on memory-constrained devices. In this paper, we proposed a new PETL framework, called Structure to Activation (S2A), to reduce the memory footprint of activation during fine-tuning. We explore model tuning by examining parametric model structures (i.e., bias, prompt, and side modules) and designing activation-efficient modules. In addition, we analyze non-parametric structures (i.e., non-linear functions) to minimize memory usage through activation quantization. Our Structure to Activation (S2A) method consequently offers a lightweight solution in terms of both parameter and memory footprint. We conduct extensive experiments on various datasets to evaluate the effectiveness of our S2A framework. The results show that our method outperforms existing PETL techniques, achieving a fourfold reduction in GPU memory footprint on average. These also demonstrate that our method is highly suitable for practical transfer learning on hardware-constrained devices.
Type
Click the Cite button above to demo the feature to enable visitors to import publication metadata into their reference management software.